The system’s functionality is derived from design goals, with specific features informed by findings from the auto-ethnographic study. In this section, we present the system setup and provide a detailed description of its functionality with a brief overview of its technical implementation.
System Setup
We built our ceramic corner with a VEVOR 9.8" LCD Touch Screen Clay Wheel GCJX-008 as the main device and Logitech C920 Webcam to capture the shape data. To ensure clear distinction between the clay and the background, the corner is wrapped in black cloth, and the basin of the pottery wheel has been removed. Our tool is built on Meta Quest3, utilizing its passthrough mode to allow users to interact with their surroundings.
The setup process is straightforward: users wear the headset, sit in front of the pottery wheel, select the appropriate speed and mode and initialize the selected tutorial using the controller. They then practice ceramic-making by hand while using voice commands to control the system’s guidance.
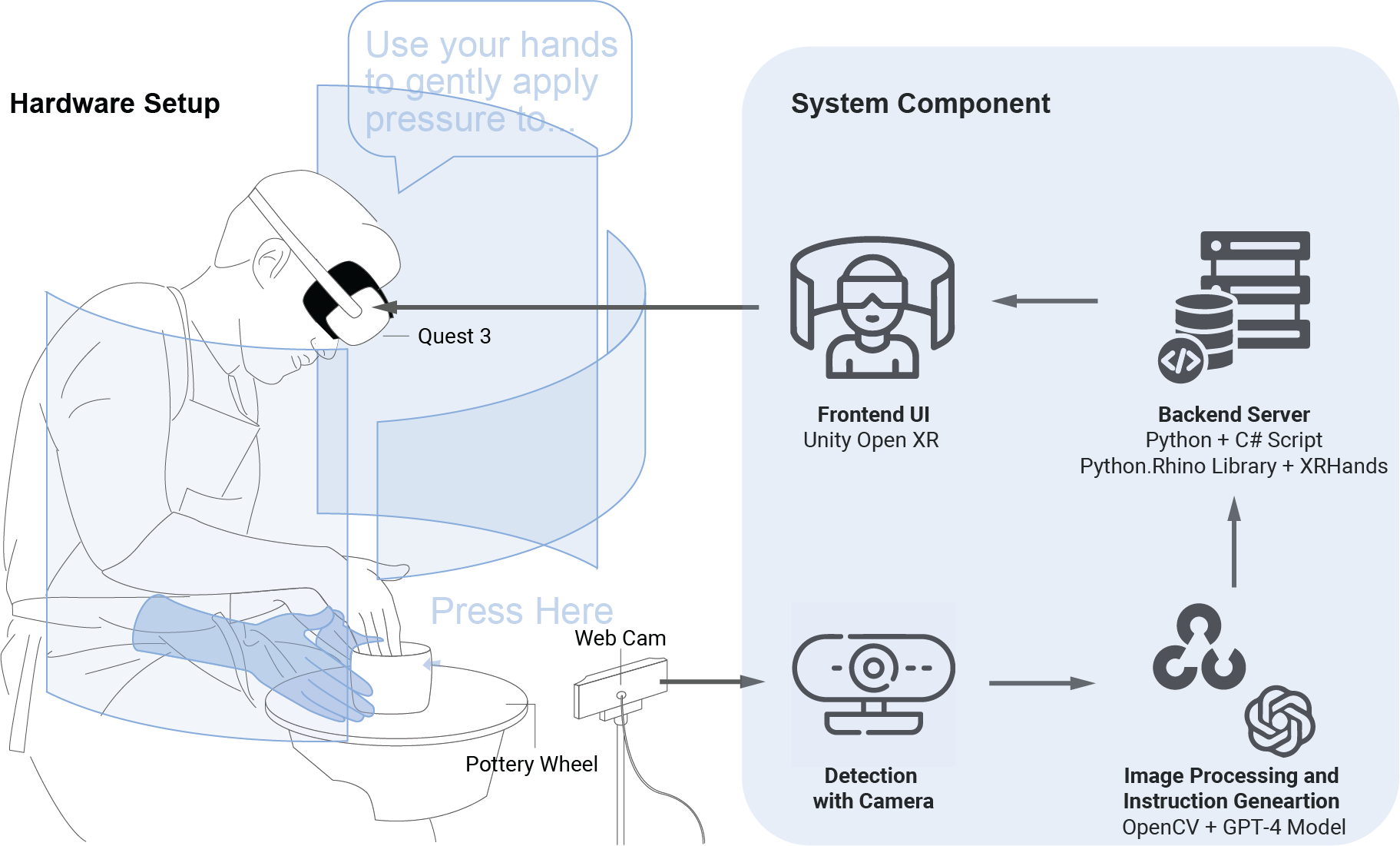
Functionality
We propose two core features in the system, customize and organize them for the two user levels according to their respective needs identified during our formative study.
Core Features
We identify two core features in our system: Hand and shape holograms as immersive tutorial and spatial-based correction, guidance, and suggestions as feedback.
Hand and Shape Holograms
Our system offers hand and shape holograms to display the first-angle view of each step and the target shape. The gesture holograms are created based on schematic diagrams, modeled in Blender, exported as .fbx animation and imported into Unity as prefabs. For gesture recognition, we piggybacked the XRHand package to visualize the gesture recognition as text-based instruction. The language is simplified to make it easier to understand, ensuring accessibility for learners of varying skill levels.
Spatial-based Feedback
Our system provides three types of feedback: correction, guidance and suggestions based on clay shape. We use the Python-based computer vision library OpenCV to capture the shape outline and identify critical positions using the Rhino.Python library. Progress is then evaluated by comparing the current shape to the target shape, generating similarity scores and boolean values based on quality rules. The evaluation results are further processed in two ways: (1) Text-based suggestions are generated using personalized prompts of different skill levels with OpenAI API based on the comparison of identified critical position locations. (2) Several profile slices are created at the intersection points between the target shape/current shape outlines, which are then swept to create spatial shape points. Both data outputs are sent to the system via servers and visualized as: (1) an overlay on the anchor with three colors: red for "push inward," green for "correct shape," and blue for "pull outward." (2) multimodal tutorial information
For Novice Learner: Step-by-step Learning
Novice learners usually have limited proficiency in ceramic making. They are less familiar with each step, lack practice for wheel-throwing techniques, and need basic feedback to improve their practice. The system provides detailed step-by-step tutorial and easy-to-understand feedback during and after the learning process.
Learning Flow: Watch, Imitate and Practice
From ethnographic study, we observed that students observe and follow their instructors, and their gestures are occasionally corrected. Correct and precise gestures are critical in wheel-throwing due to the high precision required in ceramic making. However, traditional teaching methods often struggle to provide real-time feedback on gestures during the shadowing process. Our system adopts a similar procedure but enhances the experience: users first watch a holographic animation to observe the gestures, then imitate the starting gesture with real-time hand-part suggestions and practice what they have learned from the hologram.
Spatial learning: Hologram, Video and Tips
Wheel-throwing is a three-dimensional task, requiring learners to shape clay in space. From our observation, in traditional studio settings, observation alone is often insufficient to capture the nuanced details of hand movements and their interaction with clay; even with hands-on guidance, the learner’s observation is typically limited to a third-person view. Our system leverages MR to create a spatial immersive display, providing 1) first-person hologram overlay to display the relationship between hands and clay, allowing users to closely observe and understand the intricate details of the gesture; 2) third-person demonstration videos and tips from experts to restore the knowledge from traditional studio by conveying contextual factors such as moisture, pressure, and speed. The system enhances the learner’s understanding of both explicit and tacit aspects of ceramic making, bridging the gap between traditional and immersive learning experiences.
Learn from feedback
For novice learners, it is crucial to receive feedback during their learning journey when they encounter difficulties and learn how to improve for their next attempt. Our system fulfills these needs by providing correction during ’critical incidents’, restoring the hands-on instructional experience of in-person learning. The system also generates ad-hoc summaries with suggestions for the final clay shape, allowing learners to reflect on their current attempt and improve their performance in the next round.
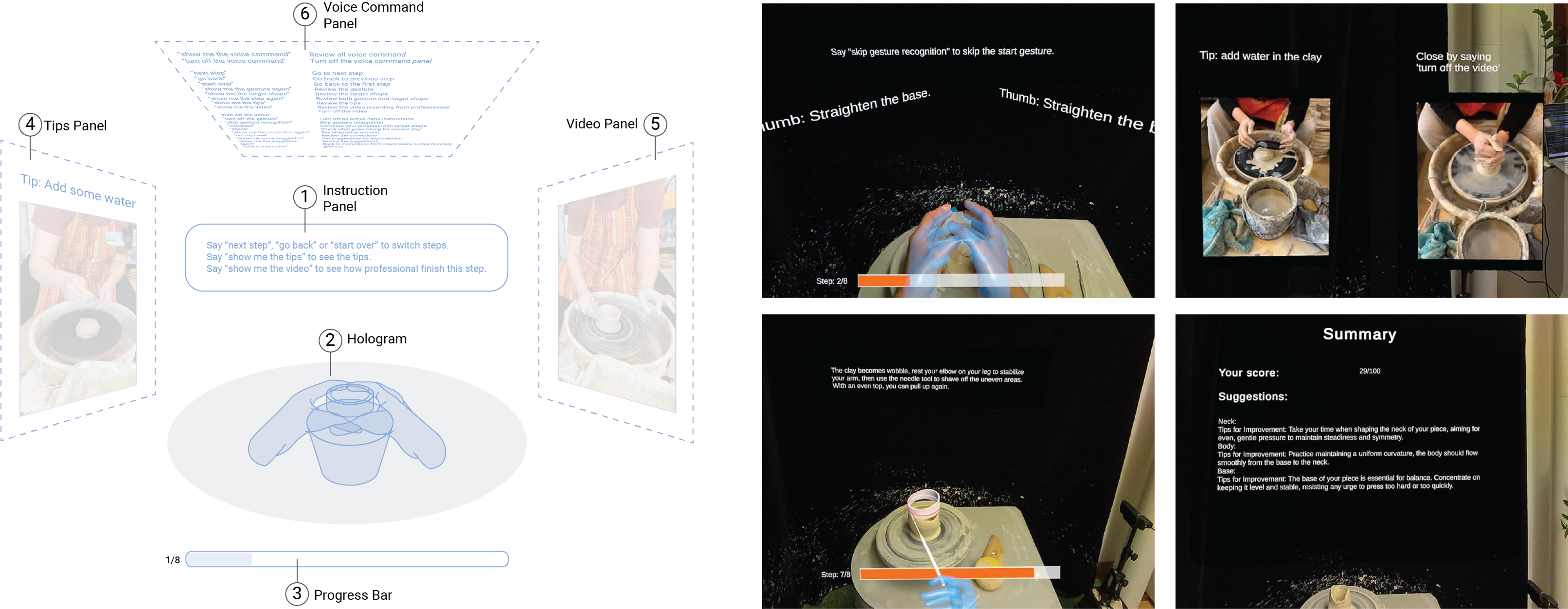
For Experienced Practitioner: Guiding and Learning
Experienced practitioners have acquired necessary skills to make ceramics but still have space for improvement. The system aims to provide them with the freedom to work independently while offering support to refine their pieces, enhancing their understanding of the craft through augmented practice.
Revisit and Reference
During our ceramic study journey and pilot studies, we observed the need among experienced practitioners to refresh their skills. They can revisit gestures and shape holograms to reinforce their techniques at any time. The system also visualizes the current and target shape in real-time on the side panel for reference, the practitioners have the flexibility to make pottery at their own pace while periodically refer to the update as needed.
Shape Guidance
For experienced practitioners, the goal shifts from simply creating a decent ceramic shape to achieving perfection. We provide real-time color-coded overlays to guide them in shaping the clay by comparing the current shape to the target shape during the process. They can refer to the overlay and fix the shape accordingly.
Multimodal Suggestion
For experienced practitioners, their advanced knowledge of skills and tools lead to higher expectations for more detailed and precise instructional assistance. Expanding on the summary in elementary mode, our system provides multimodal suggestions based on essential pottery parts, integrating text, audio, and gesture/shape hologram. This multimodal approach provides more detailed information, making it easier for experienced users to absorb and expand their knowledge.
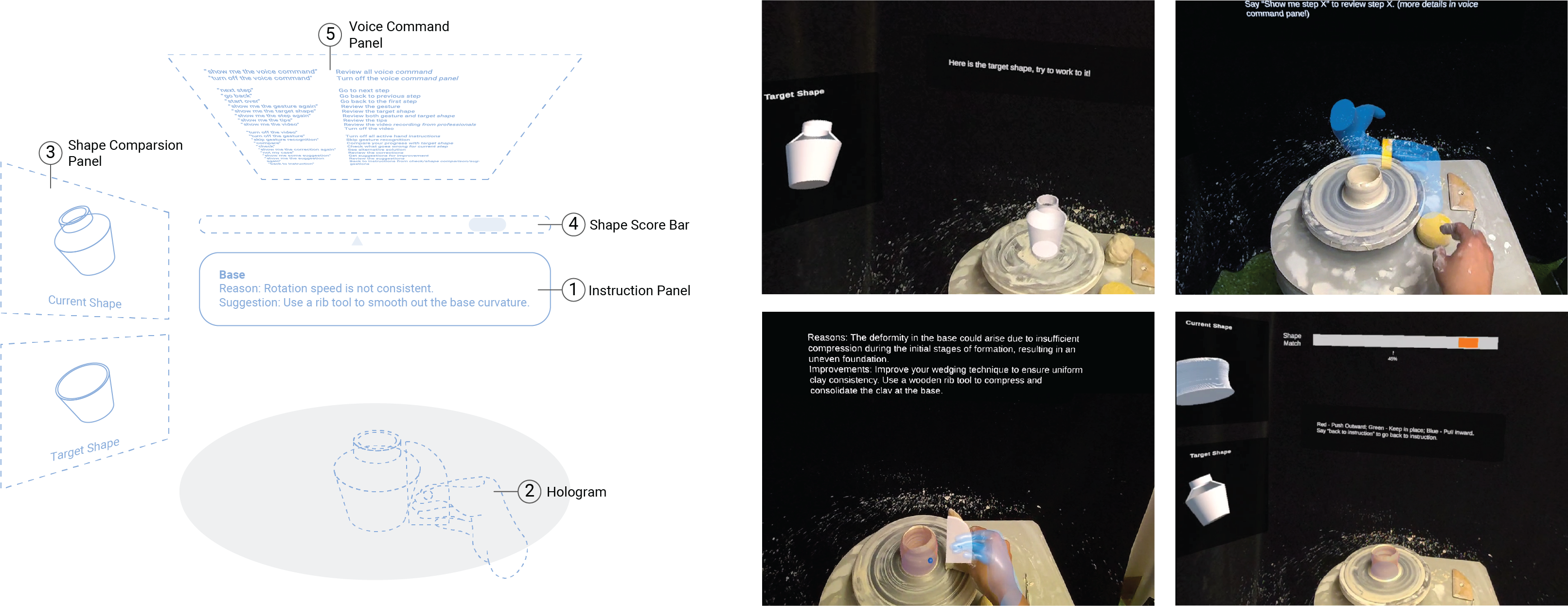